In this article we will present some key areas that affect the future of Big Data analytics, while focusing on such topics as cloud migration, the development of native data platforms for cloud environments, real-time data processing, data quality and the growing importance of "low-code" and "no-code" solutions. We will analyze how these trends are revolutionizing the way organizations store, process and use data, highlighting their key aspects and benefits. We will uncover a vision for the future of Big Data and make bold predictions that influence development in this field.
Cloud migration
Nowadays, the prevalence of companies utilizing on-premise Big Data platforms is decreasing steadily. This shift can be attributed to the numerous benefits associated with the alternative solution - cloud-based platforms, which offer cost savings and simplified implementation and management of data platforms.
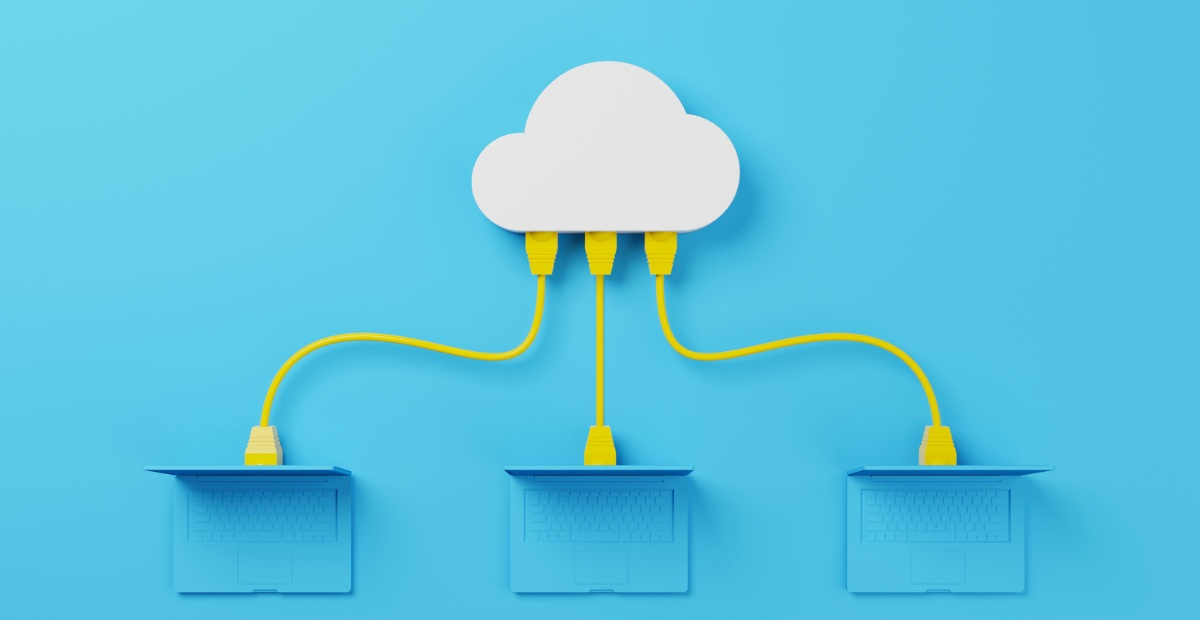
To illustrate the challenges associated with on-premise solutions, let's consider this example. Creating one from scratch involves:
- Establishing a physical server room, either by constructing a dedicated facility or renting one.
- Procuring the necessary hardware, including network equipment, storage devices, processors, and memory modules.
- Ensuring regular hardware updates and implementing robust cybersecurity systems.
As the data platform expands, additional expenses arise, such as acquiring more storage capacity to accommodate growing data set volumes and potentially upgrading computing resources. These examples fall under capital expenditures, commonly referred to as CapEx.
Moreover, maintaining an on-premise Big Data platform incurs ongoing operational expenses, known as OpEx, which encompass:
- Overseeing the upkeep of the building and server room, including energy consumption, cooling, hardware maintenance, and software licenses.
- Employing and training additional personnel, such as skilled engineers, to manage the platform effectively.
Considering the substantial financial commitments involved, both in terms of CapEx and OpEx, it becomes evident that on-premise solutions are not the most cost-effective choice for the vast majority of companies.
Therefore, migrations of Big Data platforms to the cloud are becoming increasingly common. Clouds operate on a pay-as-you-go system, which only affect OpEx costs, while simplifying the complex processes associated with maintaining an on-premise solution.
Cloud solutions also offer:
- Scalability: The ability to scale on-demand computing power, memory, and storage. As the platform grows, this is crucial in dealing with the increasing volumes of data.
- A wide range of managed and serverless data analysis and processing tools are available for various Big Data solutions: Depending on the chosen cloud service provider, big data analytical tools for batch and real-time data processing are available, leveraging technologies such as Apache Spark, Hadoop, Apache Kafka, Hive, and many more.
- Tailored storage options: Cloud storage is well-suited to the needs of Big Data. As Big Data applications often process files from different time periods, the type of storage can be customized accordingly. Frequently accessed data sets can be stored on faster disks, while less frequently used data can reside on slower, more cost-effective storage solutions.
- Security and resilience: Cloud providers invest significant resources in data security to ensure constant availability. This is a vital aspect of cloud computing, ensuring that data remains protected and always available, even in the event of breakdowns.
These are just a few of the many advantages of migrating Big Data platforms to the cloud. Clouds significantly simplify the creation and development of Big Data applications while optimizing costs according to specific needs.
Cloud-native data platforms
Cloud-native data platforms are powerful systems that are revolutionizing the way huge amounts of data are stored and analyzed in cloud environments. They enable companies to reap the maximum benefits of cloud capabilities, while reducing costs and accelerating the implementation of solutions for data-driven organizations.
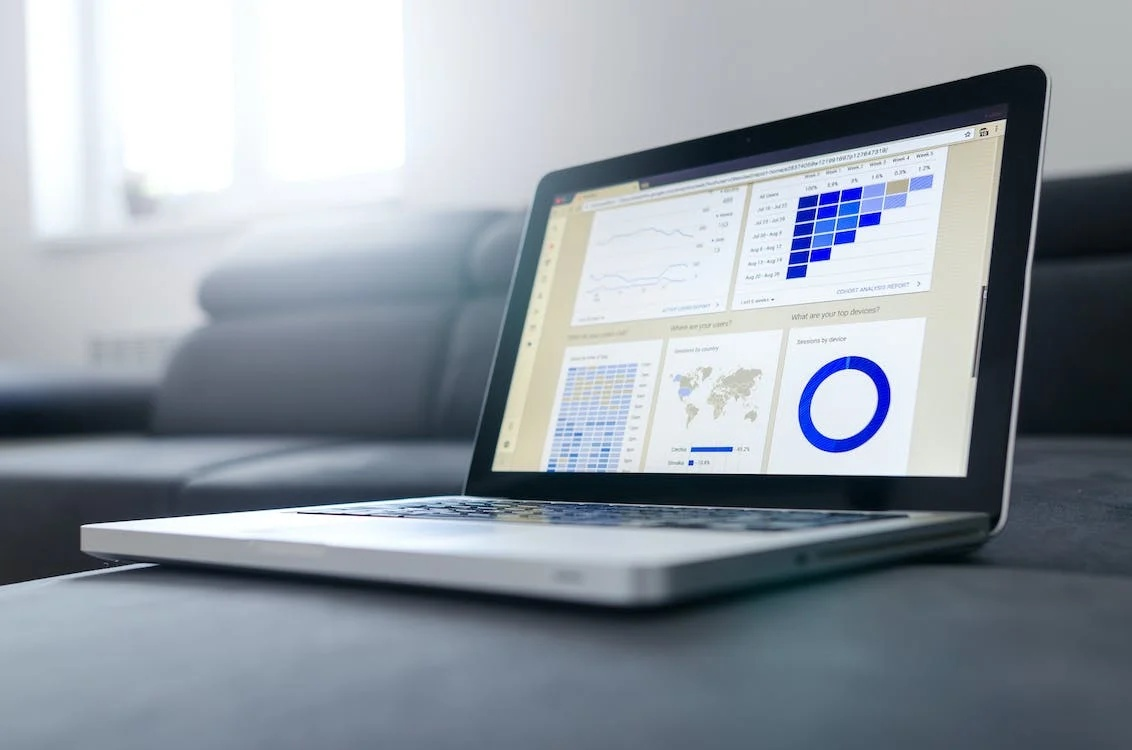
One of the key strengths of cloud-native platforms is their flexibility and scalability. As a result, users can easily adapt cloud resources to their needs, scaling up or down. This means that organizations can efficiently utilize resources according to workloads, which translates into cost optimization.
Analytical big data tools offered by cloud-native platforms are dedicated to data processing, analysis and visualization. With them, users can generate advanced reports, draw valuable business insights and utilize machine learning and artificial intelligence techniques. This enables organizations to realize the full potential of their data and make better-informed decisions.
Security and compliance are other important features of cloud-native platforms. With built-in data encryption and access control mechanisms, users can rest assured that their data is protected. Additionally, these platforms often comply with regulations such as GDPR or PCI-DSS, which is extremely important for organizations operating in regulated industries.
Cloud-native data platforms also offer support for different data types. They can handle structured data, semi-structured and unstructured data from a wide range of sources. As a result, organizations can effectively manage and analyze a large variety of data, increasing the potential for its use.
The growth in popularity of cloud-native platforms is inherent to market needs. Statistics from insights.stackoverflow.com confirm that platforms such as Databricks, BigQuery and Snowflake are gaining recognition amongst organizations. Their advanced features, ease of use and operational efficiency are attracting more and more users who recognize the potential of these solutions in the context of data storage and analysis.
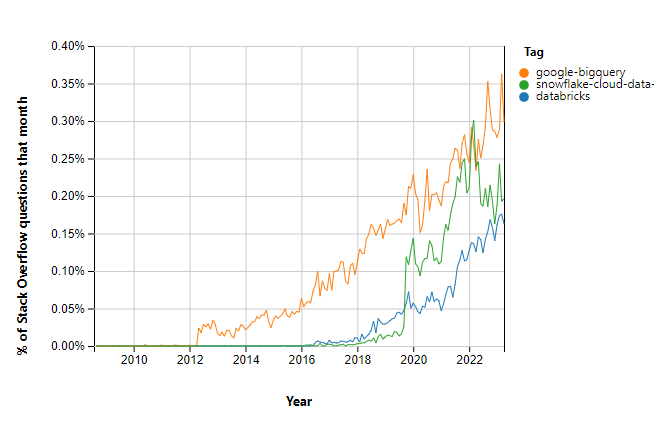
Cloud-native data platforms are revolutionizing the way organizations store and use data. With their flexibility, analytical tools, security, support for different types of data and user-frendlines, these platforms are a key element of success for organizations seeking to manage data effectively and make better-informed decisions.
Real-time data processing
Real-time data processing is playing an increasingly important role in the field of Big Data and has massive future potential. With increasing sources of big data, such as social media, IoT devices and manufacturing systems, large companies need fast and efficient analysis of their data to make real-time business decisions.
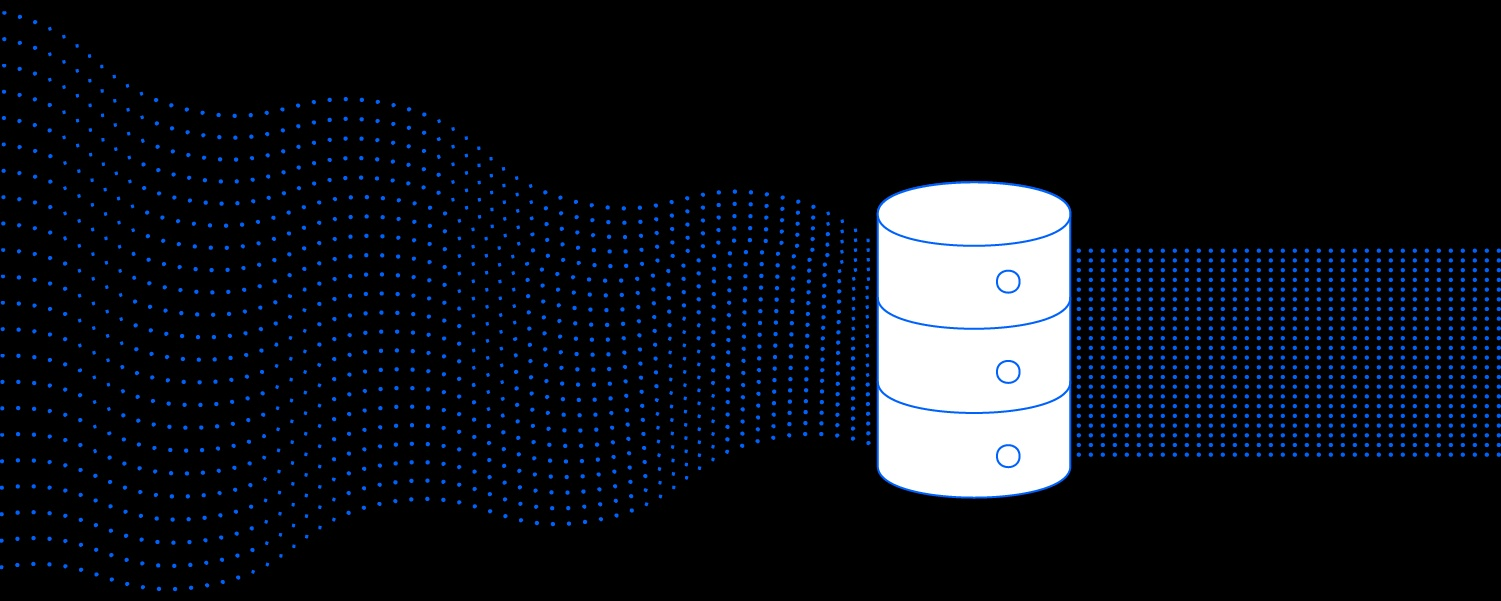
Stream processing systems integrate perfectly with data warehousing tools, data analysis tools and reporting systems. This allows data from different sources to flow seamlessly into analysis and reporting processes, providing a consistent and comprehensive view of the data.
A key advantage of stream processing is the ability to build a Big Data platform that combines streaming data with batch processing. This allows organizations to take advantage of the full range of data, both those received in real time and those collected historically. This combination provides the ability to generate even more comprehensive and precise analysis and forecasts.
One of the key strengths of stream processing systems is their scalability. They allow flexible adaptation to growing data volumes and workloads, ensuring high performance even with large data streams. This means that organizations can expand their computing and processing resources as needed, without negatively affecting system performance.
According to statistics from insights.stackoverflow.com, real-time data processing technologies are becoming increasingly popular. In particular, Apache Kafka, which is one of the leading stream processing solutions, has seen a huge raise in popularity in recent years.

The future of real-time processing in Big Data is extremely promising. With it's speed, scalability and ability to integrate with other analytical tools, stream processing systems are a key element in real-time strategic business decision-making. As technology advances and the amount of data generated increases, real-time processing will play an even bigger role in the future.
Data Quality Engineering
A Data Quality Engineer plays a vital role in ensuring the reliability and accuracy of an organization's data. Their primary responsibility is to identify, evaluate, and address any data quality issues to guarantee the integrity and consistency of the data.

The tasks performed by a Data Quality Engineer encompass a wide range of activities. They analyze and assess the quality of the data, develop and implement effective strategies for data quality control, establish robust tools and processes to enhance data quality, continuously monitor and report on the quality of data, and collaborate closely with other teams such as data analysts and software engineers.
In today's data-driven world, the demand for Data Quality Engineers is on the rise as businesses recognize the importants of reliable and high-quality data for informed decision-making, data analysis, and overall success. Incomplete or inconsistent data can lead to erroneous conclusions and detrimental choices, undermining an organization's reputation.
Considering the ever-increasing volume of data being generated by companies and the increasing reliance on data-driven decision-making, Data Quality Engineers are becoming vitally important and sought-after. With the rapid advancement of technologies like artificial intelligence, machine learning, and advanced data analytics, new challenges in maintaining data quality are constantly emerging, demanding specialized expertise and skills. Thus, Data Quality Engineering can be considered a future-oriented specialization that will continue to gain importance in the data-driven and analytics-centric landscape.
Low-code and no-code solutions
In today's fast-paced business environment, the concepts of "low-code" and "no-code" are gaining significant popularity. These approaches offer a way to develop applications and solutions quickly without extensive programming knowledge.

"Low-code" solutions streamline application development by minimizing the need for traditional coding. Developers can utilize pre-built modules and customizable tools that require minimal programming. This accelerates the development process and makes it more intuitive.
On the other hand, "no-code" takes simplicity to the next level by eliminating the need to write any code at all. "No-code" platforms provide graphical interfaces and drag-and-drop functionality, enabling individuals without deep programming expertise, such as business analysts or designers, to create their own applications.
Focusing on Big Data, "low-code" and "no-code" solutions hold tremendous potential. Big Data requires efficient tools for fast data processing and analysis. With these approaches, analysts and domain experts can create tailored tools and solutions specific to their business needs, bypassing the need for developer involvement.
The future of "low-code" and "no-code" solutions in the realm of Big Data looks promising. As these technologies continue to evolve, their functionality and flexibility will only grow. Organizations will increasingly adopt these tools to reduce project implementation time, boost team productivity, and facilitate faster data-driven decision-making.
However, it's important to note that "low-code" and "no-code" solutions will not completely replace traditional programming in every instance. For complex projects that demand specialized solutions and optimizations, coding expertise will still be necessary. Nonetheless, the rise of "low-code" and "no-code" opens up new possibilities for individuals without extensive programming knowledge, enabling them to creatively harness the power of Big Data in various business domains.
Summary
In conclusion, the future of Big Data is shaped by several key areas that are driving significant advancements in data analytics. Cloud migration offers cost savings, scalability, and a wide range of big data tools, making them an increasingly popular choice for organizations. Cloud-native data platforms revolutionize data storage and analysis in the cloud, providing flexibility, security, and support for different data types. Real-time data processing plays a crucial role in making real-time business decisions and is expected to have even greater prominence in the future. Data quality engineering ensures reliable and accurate data, which is becoming increasingly essential as volumes of data continue to grow. Lastly, low-code and no-code solutions enable users to quickly develop applications and solutions.
These areas are driving the future of Big Data, enabling organizations to optimize costs, extract valuable insights, and make better-informed decisions. As technology continues to advance, these trends will shape the way data is stored, processed, and utilized, ultimately transforming industries and creating new opportunities for innovation and growth. Embracing these big bets in the future of Big Data will be crucial for organizations seeking to stay competitive in an increasingly data-centric world.